Machine learning and optimization are revolutionizing how we solve complex problems across industries, transforming raw data into actionable intelligence. By combining advanced algorithms with unprecedented computing power, organizations are now achieving what was once thought impossible – from predicting market trends with remarkable accuracy to optimizing supply chains in real-time.
Today’s cutting-edge bioenergy innovation demonstrates how these technologies work in harmony: optimization algorithms identify the most efficient processing methods, while machine learning systems continuously adapt and improve performance based on real-world data.
The convergence of these two fields represents more than just technological advancement – it’s a fundamental shift in how we approach problem-solving. From healthcare diagnostics to renewable energy systems, the combination of optimization techniques and machine learning is creating solutions that are not just more efficient, but also more intelligent and adaptable than ever before.
As we stand at the intersection of big data and artificial intelligence, understanding these technologies isn’t just advantageous – it’s essential for staying competitive in an increasingly data-driven world.
Smart Algorithms Transform Biomass Processing
Real-time Process Optimization
Machine learning algorithms are revolutionizing biomass conversion processes by enabling real-time optimization that would have been impossible just a few years ago. These smart systems continuously monitor and adjust multiple parameters simultaneously, creating a dynamic feedback loop that maximizes efficiency and output.
In leading Australian bioenergy facilities, ML algorithms analyze data from sensors throughout the conversion process, making split-second decisions to optimize conditions. For example, at a facility in Victoria, smart systems monitor factors like temperature, pressure, and moisture content, automatically adjusting settings to maintain ideal conditions for maximum yield.
The real-world impact is significant. These systems have demonstrated improvements in biomass conversion efficiency by up to 25%, while reducing waste products by nearly 40%. When processing agricultural residues like sugarcane bagasse or forest waste, the algorithms can adapt to variations in feedstock quality, ensuring consistent output despite changing input conditions.
What makes these systems particularly valuable is their ability to learn and improve over time. As they process more data, they become increasingly adept at predicting optimal conditions for different types of biomass. This learning capability means that facilities can handle a wider variety of feedstock materials without compromising efficiency.
For facility operators, this translates to reduced operational costs and improved sustainability metrics. The systems can even predict maintenance needs before equipment failures occur, minimizing downtime and extending the life of critical components.
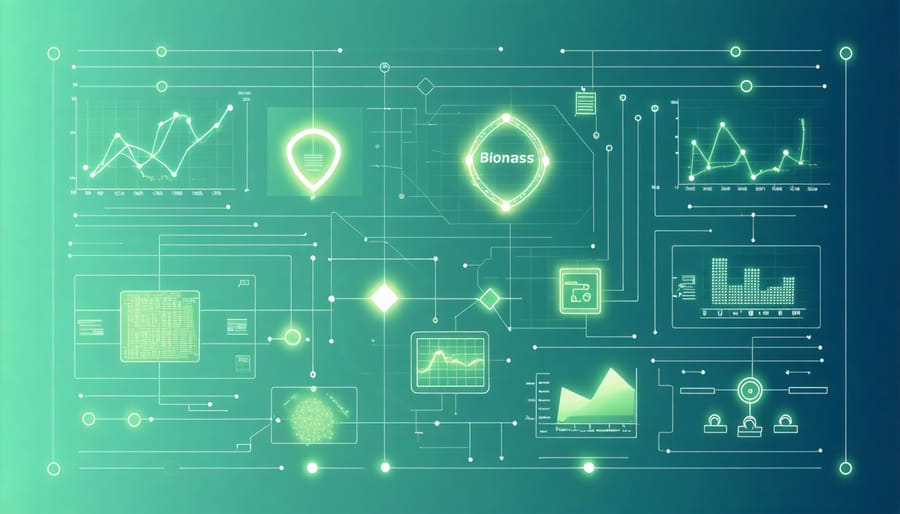
Predictive Maintenance Systems
In the dynamic landscape of modern industry, predictive maintenance systems powered by machine learning are revolutionizing how we care for equipment and infrastructure. These intelligent systems analyze real-time data from sensors and historical performance records to forecast potential failures before they occur, saving both time and resources.
Australian mining companies have been early adopters of this technology, with success stories like the Rio Tinto operations in the Pilbara region. Their automated systems continuously monitor thousands of data points across their heavy machinery fleet, predicting maintenance needs with remarkable accuracy and reducing unplanned downtime by up to 75%.
Machine learning algorithms excel at identifying subtle patterns that might escape human observation. For instance, they can detect minute changes in equipment vibration, temperature, or power consumption that signal impending issues. This proactive approach allows maintenance teams to schedule repairs during planned downtimes, maximizing operational efficiency.
The benefits extend beyond just preventing breakdowns. These systems optimize maintenance schedules by determining the perfect balance between too frequent and too infrequent servicing. A Queensland manufacturing plant reported a 30% reduction in maintenance costs after implementing ML-based predictive maintenance, while also extending their equipment’s lifespan.
Looking ahead, these systems are becoming more sophisticated, incorporating environmental factors and operational contexts to provide increasingly accurate predictions. As the technology evolves, it’s making maintenance more sustainable by reducing waste and optimizing resource use.
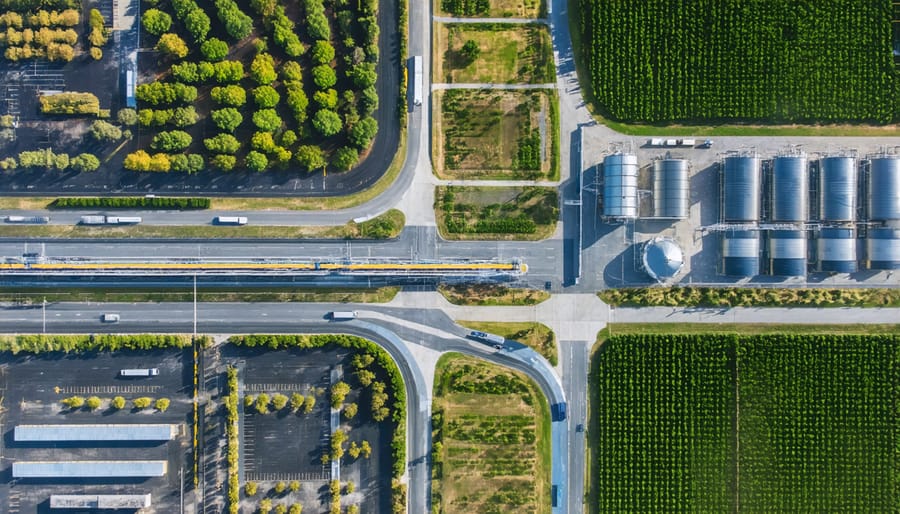
Machine Learning in Biomass Supply Chain Management
Smart Feedstock Selection
In the evolving landscape of bioenergy production, artificial intelligence is revolutionising how we select and evaluate feedstock materials. Smart algorithms now analyse vast datasets of biomass characteristics, helping facility operators make data-driven decisions about which materials will yield the best results.
Australian bioenergy facilities are increasingly adopting AI-powered systems that can assess multiple feedstock parameters simultaneously. These systems evaluate factors like moisture content, energy density, availability, and transportation costs to identify the most efficient combinations of biomass sources.
For instance, in Queensland’s sugar-growing regions, AI systems help processing facilities determine the optimal mix of bagasse, wood waste, and agricultural residues. The technology considers seasonal variations, storage requirements, and processing capabilities to maintain consistent energy production throughout the year.
Machine learning algorithms are particularly valuable in predicting feedstock quality and potential yields. By analysing historical data and real-time measurements, these systems can forecast how different biomass sources will perform under various processing conditions. This predictive capability helps operators adjust their feedstock selection strategies to maximise output while minimising waste and operational costs.
The technology also helps identify unexpected synergies between different biomass sources. For example, some facilities have discovered that mixing urban green waste with agricultural residues can improve overall conversion efficiency, a finding that wouldn’t have been obvious without AI-powered analysis.
Looking ahead, these smart selection systems are becoming more sophisticated, incorporating weather data, market prices, and sustainability metrics to optimise not just operational efficiency, but also environmental outcomes and economic benefits.
Logistics Optimization
Machine learning is revolutionizing logistics optimization in the bioenergy sector, creating smarter and more efficient supply chains across Australia. By analyzing vast amounts of data from transport routes, storage facilities, and delivery schedules, AI-powered systems are helping organizations reduce costs while minimizing their environmental footprint.
Advanced algorithms now optimize delivery routes by considering multiple variables simultaneously, including fuel consumption, vehicle capacity, and time constraints. These systems can adapt in real-time to changing conditions, such as weather events or road closures, ensuring minimal disruption to operations. For instance, major biomass processors in Queensland have reported up to 25% reduction in transport costs after implementing ML-based routing systems.
Storage optimization has also seen significant improvements through predictive analytics. Smart warehousing systems can forecast demand patterns and adjust inventory levels accordingly, reducing waste and ensuring optimal storage conditions for different types of biomass materials. This technology has proven particularly valuable for managing seasonal variations in biomass availability.
The integration of IoT sensors with machine learning has enhanced bioenergy supply chain transparency and efficiency. These systems monitor storage conditions, track shipment locations, and predict maintenance needs before equipment failures occur.
Looking ahead, emerging technologies like autonomous vehicles and drone delivery systems are being tested in various pilot programs across the country. These innovations promise to further streamline logistics operations while reducing the carbon footprint of bioenergy transportation. The combination of AI-driven optimization and autonomous systems represents the next frontier in sustainable logistics management.
AI-Driven Plant Operation and Control
Automated Process Control
Modern bioenergy facilities are embracing AI-driven process control systems to optimize their operations like never before. These intelligent systems continuously monitor and adjust various parameters in real-time, ensuring peak performance while maintaining sustainability goals.
At facilities across Australia, smart sensors collect data on temperature, pressure, feedstock quality, and conversion rates. Machine learning algorithms process this information to make split-second decisions, maintaining optimal conditions throughout the entire production process. For instance, the Ballarat Bioenergy Plant uses automated controls to adjust air flow and feedstock input rates, resulting in a 15% increase in energy output while reducing emissions.
The beauty of automated process control lies in its ability to learn and adapt. As these systems gather more operational data, they become increasingly efficient at predicting and preventing potential issues before they occur. This predictive capability has helped facilities reduce downtime by up to 30% and cut maintenance costs significantly.
These systems also excel at balancing multiple objectives simultaneously – maximizing energy production, minimizing emissions, and ensuring consistent output quality. For plant operators, this means less time spent on routine adjustments and more focus on strategic improvements.
The integration of automated controls has proven particularly valuable during challenging weather conditions or unexpected feedstock variations, maintaining stable operations when manual control might struggle to keep up.
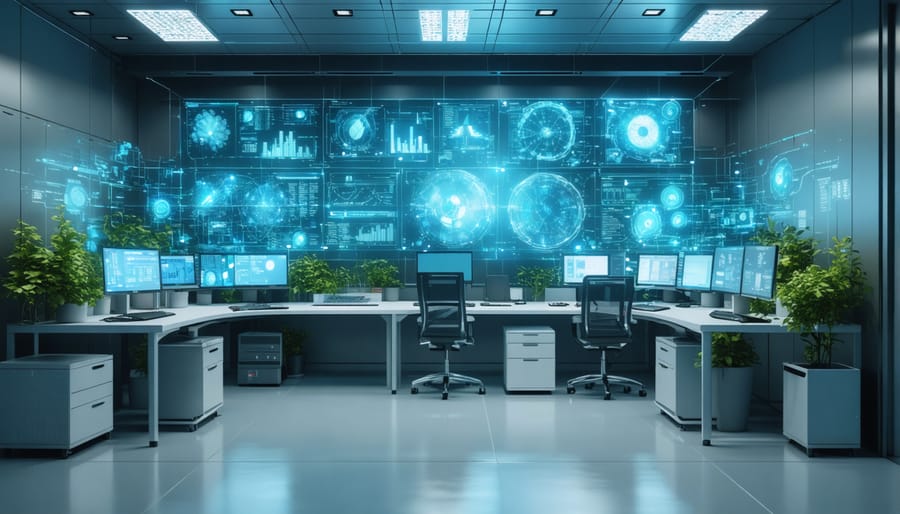
Energy Output Optimization
Machine learning is revolutionizing how we optimize energy output in renewable systems, particularly in Australia’s growing bioenergy sector. By analyzing vast amounts of operational data, AI algorithms can predict optimal conditions for energy production and automatically adjust system parameters in real-time.
These smart systems are particularly effective in bioenergy grid integration, where they monitor multiple variables simultaneously – from feedstock quality to weather conditions – to maximize energy yield while minimizing waste. For instance, at the Yarwun Bioenergy Facility in Queensland, machine learning algorithms have helped increase energy output by 15% through precise control of anaerobic digestion processes.
The technology also excels at predictive maintenance, identifying potential equipment failures before they occur and scheduling maintenance during low-demand periods. This proactive approach has reduced downtime by up to 30% in several Australian facilities.
Real-world applications include smart feedstock management systems that optimize storage conditions and processing timing, and intelligent load balancing that ensures steady power supply despite varying biomass availability. These systems learn from historical data to make increasingly accurate predictions about energy demand patterns and adjust production accordingly.
Looking ahead, emerging AI technologies promise even greater efficiencies through advanced pattern recognition and autonomous decision-making capabilities, positioning Australia’s bioenergy sector for sustainable growth and improved reliability.
Future Applications and Australian Innovation
As Australia continues to embrace technological advancement, the intersection of artificial intelligence and bioenergy optimization presents exciting opportunities for our sustainable future. Leading research institutions across the country are developing sophisticated renewable energy innovations that promise to revolutionize how we harness and distribute bioenergy resources.
The CSIRO’s Data61 is pioneering predictive algorithms that can forecast biomass availability with unprecedented accuracy, helping farmers and energy producers optimize their harvest and production schedules. These smart systems are being integrated with weather pattern analysis to maximize yield while minimizing environmental impact.
Several Australian startups are developing AI-powered microgrids that can automatically balance energy distribution between multiple renewable sources, including bioenergy facilities. These systems learn from usage patterns and adapt in real-time, ensuring consistent power supply while reducing waste and operational costs.
In Queensland, researchers are exploring the potential of quantum computing to solve complex optimization problems in bioenergy production. This cutting-edge technology could dramatically improve processing efficiency and reduce the carbon footprint of existing facilities.
The future of waste management is being transformed through smart sorting systems that use computer vision and machine learning to identify and separate organic materials more effectively. These innovations are particularly valuable for regional communities, where efficient waste-to-energy conversion is crucial for sustainable development.
Looking ahead, we can expect to see the emergence of fully autonomous bioenergy facilities that optimize every aspect of production, from feedstock selection to energy distribution. These systems will incorporate advanced sensors, Internet of Things (IoT) devices, and artificial intelligence to create self-managing energy networks that respond to community needs while maintaining environmental balance.
Australian innovation in this space isn’t just about technology – it’s about creating practical solutions that work for our unique landscape and communities. By combining our technological expertise with our commitment to sustainability, we’re building a future where smart bioenergy systems play a central role in our energy mix.
As we look to the future, the integration of artificial intelligence and machine learning in bioenergy optimization represents a game-changing shift in Australia’s renewable energy landscape. These technologies have already demonstrated their ability to significantly improve efficiency, reduce costs, and maximize output across the bioenergy sector.
The impact is particularly evident in our success stories, such as the innovative waste-to-energy facilities in Western Australia that have achieved up to 30% better performance through AI-driven optimization. These achievements showcase how smart technologies are helping Australia move closer to its renewable energy targets while creating sustainable solutions for waste management.
Looking ahead, we can expect even more revolutionary developments. Advanced machine learning algorithms will continue to evolve, enabling more precise biomass feedstock selection, improved process control, and enhanced energy output prediction. The integration of real-time monitoring systems with AI will create increasingly autonomous and efficient bioenergy facilities, making renewable energy more accessible and affordable for all Australians.
The future also holds promise for smaller-scale applications, with AI-powered systems becoming more compact and cost-effective for regional communities. This democratization of bioenergy technology will play a crucial role in Australia’s transition to a more sustainable energy future.
As we embrace these technological advances, the partnership between human expertise and artificial intelligence will remain key to unlocking the full potential of bioenergy resources, ensuring a cleaner, greener tomorrow for generations to come.